My First Knowledge Graph
This tutorial is designed to give users their first introduction to the concept of a knowledge graph.
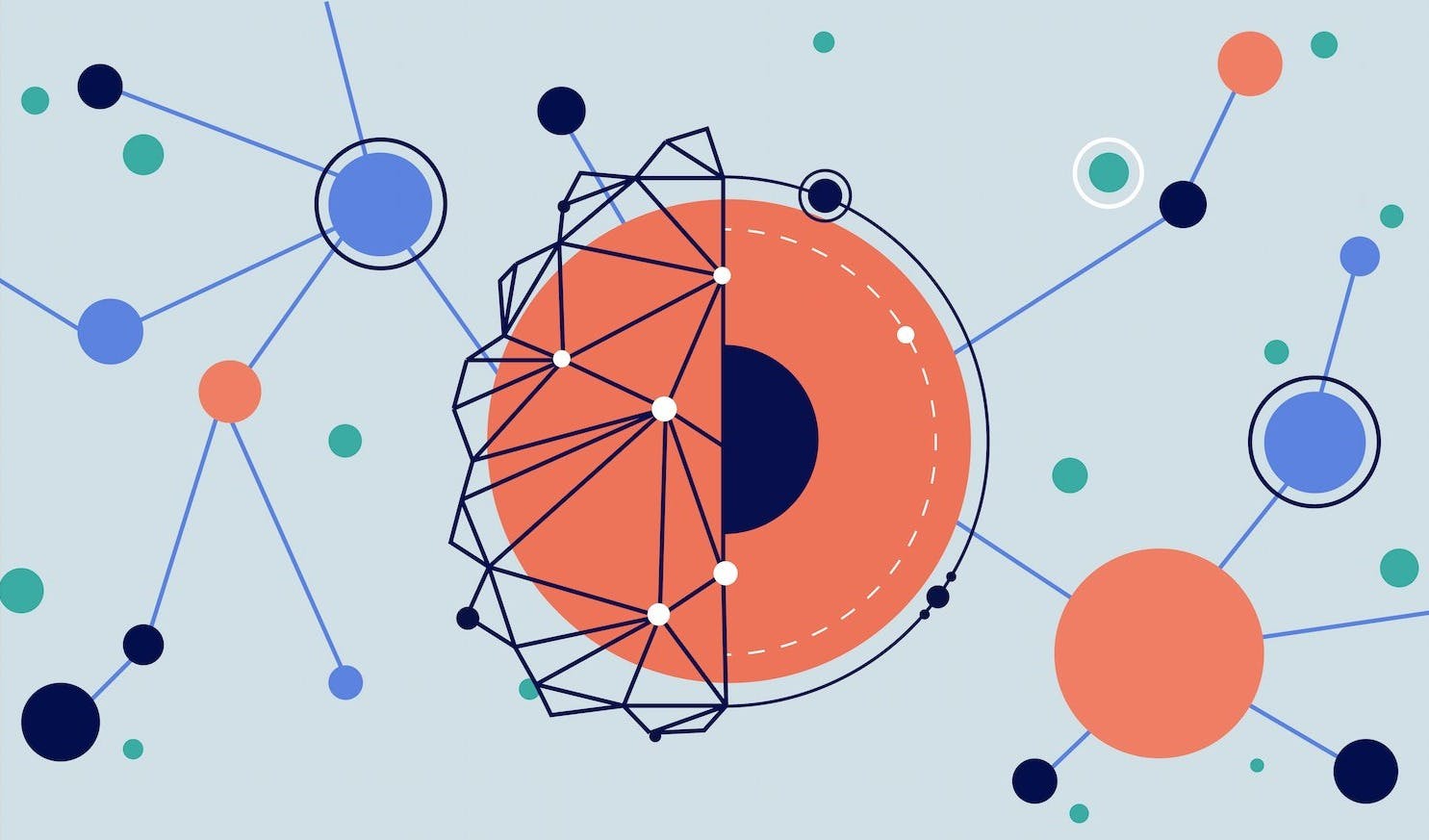
Goal
The goal of this tutorial is to introduce the concept of a knowledge graph and practice some simple Rel that will allow you to create your first knowledge graph.
Scenario
Imagine that you want to analyze the performances of different Olympic athletes to find the most successful athlete. Success can be measured in various different ways. You have two questions to ask about your data:
- Which athlete participated in the most games?
- Which athletes participated in both Winter and Summer games?
You can now begin to explore how to model this domain as a knowledge graph and how to express these questions as graph queries.
Elements of a Knowledge Graph
Knowledge graphs allow you to organize information and define relationships between entities. If you think about the Olympic Games scenario, you can intuitively identify two major concepts: Olympic Games and athletes. They are referred to as “entity nodes” and are the solid bordered nodes on the Object-Role Modeling (ORM) diagram below.
These entities can have information associated with them, such as name, age, etc. Related information like this is modeled as a “value node”, and these are represented with dashed borders.
The relationships between concepts (for example, an athlete’s Olympics participation) and the relationship between concepts and values (for example, the athlete’s name) are modeled as edges that connect the nodes with each other in the knowledge graph. In ORM diagrams, edges are represented as the series of boxes connecting the nodes.
ORM diagrams are powerful tools to express and visualize complex data models at a conceptual level. Rel knowledge graphs and ORM diagrams support advanced representation of data such as hyperedges (edges between more than two nodes). You will find them throughout this tutorial illustrating your knowledge graph as it grows.
Here’s a summary of everything you just learned about knowledge graphs:
Term | Description |
---|---|
Entity node | Main concepts. For example: athlete. |
Value node | Information related to an entity node. For example: athlete’s age. |
Edge | The relationship between two nodes. |
You can find a more advanced version of this table in Elements of a Relational Knowledge Graph.
To learn more about how entities and value types can be constructed in Rel, see Entities and Value Types. Note: This tutorial uses a simplified construction of entities and values.
Modeling Your Knowledge Graph in Rel
You can build a model of your data by describing nodes and the edges between them. By giving these nodes and edges meaning, you are turning a graph into a knowledge graph.
Here is an ORM diagram of the knowledge graph you are about to build:
ORM diagrams allow you to give a visual representation of the categories of information you have in your knowledge graph such as “Olympics”. They are not used to represent specific data, such as “Tokyo 2020”.
Structuring the Data
The easiest way to start is by listing some things that you know. You have information regarding:
- The Olympic Games (its name, the city and year they occurred, and the type of game it is).
- The athletes (their names and the sport(s) they performed in).
- The athletes’ participation in the Olympic Games.
Listing what you know is called defining the graph schema. You can find more details and examples of schemas in Elements of a Relational Knowledge Graph and in Graph Schema.
By defining the schema, you will list the entity nodes as well as the value nodes as follows:
// model
entity type Olympics = String
entity type Athlete = String
value type Sport = String
value type Name = String
value type City = String
value type GameType = "Summer"; "Winter"
Below is a visual representation of your schema. The entity nodes have solid borders and the value nodes have dashed borders:
Note that entity types are used for entity nodes and value types are used for value nodes.
By writing entity type Olympics = String
you declare that each Olympics is identified by a single string value.
Similarly, by writing value type Sport = String
you declare that Sport is modeled as a value type containing a single string.
Both entity and value type declarations create a constructor relation, which starts with a caret ^
, that will be used to create the actual node instances.
Choosing what is an entity node and what is a value node is up to the author. You can find more information in Elements of a Relational Knowledge Graph.
It is not necessary to define the value node Year
because Year
is a value type that is already defined by the system via the Standard Library.
Populating the Graph
Now that you have defined the schema, you can insert some data into your database. It is best practice to organize data with modules and group information together. When building a knowledge graph, it makes sense to insert all the data into a module where the module represents the knowledge graph.
First, the Olympics
data gets inserted, then the Athlete
, and finally the athlete’s participation in the Olympic Games.
Updating the data within the module requires two steps:
- Defining the data within a temporary module (
olympic_game_info
in this case). - Storing the data in a base relation (
OlympicGraph
in this case) that persists in the database.
You will learn more about modules in later tutorials, but for now it’s helpful to get used to organizing your code with them.
Olympic Games
First, define an entity node Olympics
that describes Olympic Games and the edges (has_name
, hosted_in
, happens_in
, and has_type
) that relate the entity node to all its value nodes (Name
, City
, Year
, and Game Type
).
// write query
// Define the data within a temporary `olympic_game_info` module.
module olympic_game_info
// Entity node: `Olympics`
def Olympics = {
^Olympics["Tokyo 2020"];
^Olympics["Rio 2016"];
^Olympics["London 2012"];
^Olympics["Beijing 2008"];
^Olympics["Athens 2004"];
^Olympics["PyeongChang 2018"];
^Olympics["Sochi 2014"]
}
// Edge: `has_name`
def has_name = {
(^Olympics["Tokyo 2020"], ^Name["Tokyo 2020"]);
(^Olympics["Rio 2016"], ^Name["Rio 2016"]);
(^Olympics["London 2012"], ^Name["London 2012"]);
(^Olympics["Beijing 2008"], ^Name["Beijing 2008"]);
(^Olympics["Athens 2004"], ^Name["Athens 2004"]);
(^Olympics["PyeongChang 2018"], ^Name["PyeongChan 2018"]);
(^Olympics["Sochi 2014"], ^Name["Sochi 2014"])
}
// Edge:`hosted_in`
def hosted_in = {
(^Olympics["Tokyo 2020"], ^City["Tokyo"]);
(^Olympics["Rio 2016"], ^City["Rio de Janeiro"]);
(^Olympics["London 2012"], ^City["London"]);
(^Olympics["Beijing 2008"], ^City["Beijing"]);
(^Olympics["Athens 2004"], ^City["Athens"]);
(^Olympics["PyeongChang 2018"], ^City["PyeongChang"]);
(^Olympics["Sochi 2014"], ^City["Sochi"])
}
// Edge: `happens_in`
def happens_in = {
(^Olympics["Tokyo 2020"], ^Year[2021]);
(^Olympics["Rio 2016"], ^Year[2016]);
(^Olympics["London 2012"], ^Year[2012]);
(^Olympics["Beijing 2008"], ^Year[2008]);
(^Olympics["Athens 2004"], ^Year[2004]);
(^Olympics["PyeongChang 2018"], ^Year[2018]);
(^Olympics["Sochi 2014"], ^Year[2014])
}
// Edge: `has_type`
def has_type = {
(^Olympics["Tokyo 2020"], ^GameType["Summer"]);
(^Olympics["Rio 2016"], ^GameType["Summer"]);
(^Olympics["London 2012"], ^GameType["Summer"]);
(^Olympics["Beijing 2008"], ^GameType["Summer"]);
(^Olympics["Athens 2004"], ^GameType["Summer"]);
(^Olympics["PyeongChang 2018"], ^GameType["Winter"]);
(^Olympics["Sochi 2014"], ^GameType["Winter"])
}
end
// Store the data in the `OlympicGraph` base relation.
def insert:OlympicGraph = olympic_game_info
As you can see, a module is started with the keyword module
and closed with the keyword end
.
The indentation displayed is designed for readability purposes.
It is not required by Rel.
So far, you have specified facts about recent Olympic Games and stored these in the OlympicGraph
base relation.
Notice that you have reused ^Olympics["Tokyo 2020"]
in several edge definitions.
These edges associate the ^Olympics["Tokyo 2020"]
entity node with different value nodes attributed to that particular Olympic Game.
You can visualize the entity and value nodes as well as their properties, which appear as edges, in the ORM diagram:
Athletes
Next, you need to add the Athlete
information to the database.
Although in the real world there are thousands of Olympic athletes, for illustration purposes you are going to use a short list.
// write query
// Define the data within a temporary `olympic_game_info` module.
module olympic_game_info
// Entity node: `Athlete`
def Athlete = {
^Athlete["Allyson Felix"];
^Athlete["Eddy Alvarez"];
^Athlete["Tom Daley"];
^Athlete["Simone Biles"]
}
// Edge: `has_name`
def has_name = {
(^Athlete["Allyson Felix"], ^Name["Allyson Michelle Felix"]);
(^Athlete["Eddy Alvarez"], ^Name["Eduardo Cortes Alvarez"]);
(^Athlete["Tom Daley"], ^Name["Thomas Robert Daley"]);
(^Athlete["Simone Biles"], ^Name["Simone Arianne Biles"])
}
// Edge: `performs_in`
def performs_in = {
(^Athlete["Allyson Felix"], ^Sport["Athletics"]);
(^Athlete["Eddy Alvarez"], ^Sport["Baseball"]);
(^Athlete["Eddy Alvarez"], ^Sport["Short track speed skating"]);
(^Athlete["Tom Daley"], ^Sport["Diving"]);
(^Athlete["Simone Biles"], ^Sport["Gymnastics"])
}
end
// Store the data in the `OlympicGraph` base relation.
def insert:OlympicGraph = olympic_game_info
Again, the Rel module syntax helps you organize your thinking around modeling with easy-to-recognize blocks. The module includes definitions of names and sports for each athlete.
Note: Here the temporary module is called olympic_game_info
again.
The previous use of it and the definitions it encapsulated were erased after the storing transaction.
Only the information stored in the base relation OlympicGraph
persist in the database.
Some athletes may play in multiple sports. Eddy Alvarez, for example, competed in both baseball and short track speed skating.
But this fact doesn’t change how you specify the attribute ^Sport
.
In the case of athletes who have competed in multiple sports, you have to define one edge for each sport that they performed in.
You have specified facts for two entity nodes: Olympics
and Athlete
(solid bordered).
Notice how your knowledge graph is growing as you define more and more entity nodes and link them to their values through properties, which are modeled by edges:
Participation
Now that you have defined all your entity nodes and related value nodes, you want to model athlete participation in Olympic Games as this is the relationship you are interested in.
To do so, you write a relation called participates_in
that captures athlete participation. This relation creates the edges in your knowledge graph that connect athlete nodes with the relevant Olympic Game nodes.
Once again, you use the module syntax.
// write query
// Define the data within a temporary `olympic_game_info` module.
module olympic_game_info
// Edge: `participates_in`
def participates_in = {
(^Athlete["Eddy Alvarez"], ^Olympics["Tokyo 2020"]);
(^Athlete["Eddy Alvarez"], ^Olympics["Sochi 2014"]);
(^Athlete["Allyson Felix"], ^Olympics["Tokyo 2020"]);
(^Athlete["Allyson Felix"], ^Olympics["Rio 2016"]);
(^Athlete["Allyson Felix"], ^Olympics["London 2012"]);
(^Athlete["Allyson Felix"], ^Olympics["Beijing 2008"]);
(^Athlete["Allyson Felix"], ^Olympics["Athens 2004"]);
(^Athlete["Tom Daley"], ^Olympics["Tokyo 2020"]);
(^Athlete["Tom Daley"], ^Olympics["Rio 2016"]);
(^Athlete["Tom Daley"], ^Olympics["London 2012"]);
(^Athlete["Tom Daley"], ^Olympics["Beijing 2008"]);
(^Athlete["Simone Biles"], ^Olympics["Tokyo 2020"]);
(^Athlete["Simone Biles"], ^Olympics ["Rio 2016"])
}
end
// Store the data in the `OlympicGraph` base relation.
def insert:OlympicGraph = olympic_game_info
As you visualize this update in the ORM diagram, notice that the edges between the entity nodes look the same as the edges between entity and value nodes:
Querying Your Knowledge Graph in Rel
Start Querying
When the two entities Olympics
and Athlete
are related, you can find additional insight by asking questions — queries — about specific entity properties as well as connections between entities.
For example, you can find a collection of athletes who have participated in the “Tokyo 2020” Olympic Games.
But with the Sport
value node conveniently linked with the Athlete
entity node by the edge performs_in
, you can refine your query to find only athletes performing gymnastics in the “Tokyo 2020” Olympic Games.
// read query
def output(person, person_name) = {
OlympicGraph:performs_in(person, ^Sport["Gymnastics"])
and OlympicGraph:participates_in(person, ^Olympics["Tokyo 2020"])
and OlympicGraph:has_name(person, person_name)
}
Here’s the breakdown of that query:
def output(person, person_name)
indicates that the output will display two values: an entity hash, which is represented by the variableperson
, and the name of an athlete, which is represented by the variableperson_name
.OlympicGraph:performs_in(person, ^Sport["Gymnastics"])
selects edges that associate a person with the sport “Gymnastics”. This information will be retrieved from the moduleOlympicGraph
.and OlympicGraph:participates_in(person, ^Olympics["Tokyo 2020"])
refines this selection to gymnasts that competed in the “Tokyo 2020” Olympic Games. This information will be retrieved from the moduleOlympicGraph
.and OlympicGraph:has_name(person, person_name)
connects the entity hash with the name of the athlete.
When you first defined the schema of your database, you established that Olympics
and Athlete
are modeled as entities.
The way to reference entities is by their unique system-generated number, called a “hash”.
If you want to display the name of the athlete (person_name
) rather than its unique hash number, you need to specify it in the query.
This example represents one “hop” on a knowledge-graph, where you start at the Olympics
node and jump via the edge participates_in
to all neighboring nodes of type Athlete
that fulfill your specific condition. This type of query is characteristic of working with knowledge graphs.
Most Experienced Athlete
You can now return to your scenario and apply your knowledge of queries. You want to find:
- Athletes in your list who have participated in the most Olympic Games.
- Athletes who have participated in both Summer and Winter Olympics Games.
You can use the information in the base relation OlympicGraph
to find the most appropriate athletes from your data.
// read query
def experience[person] = count[olympics : OlympicGraph:participates_in(person, olympics)]
def most_experienced_athlete = argmax[experience]
def output(person, person_name) = {
most_experienced_athlete(person)
and OlympicGraph:has_name(person, person_name)
}
To solve the first question, you first define a list of athletes coupled with the number of Olympic Games they’ve participated in. You will call this relation experience
.
Here, you can use a built-in relation called count
to aggregate over olympics
, counting the number of Olympic Games per athlete.
Then building on the experience
definition, you can use another built-in relation called argmax
to define another relation called most_experienced_athlete
.
This function chooses from the list the athlete associated with the largest number of Olympic Games, which is stored in the relation experience
.
Finally, using the relation most_experienced_athlete
, you can query your database.
Notice that the relation most_experienced_athlete
is not defined within the base relation OlympicGraph
and therefore referencing it doesn’t require the OlympicGraph:
prefix.
Furthermore, the relation only exists within this query and is not persisted in the database and can’t be accessed elsewhere.
Multi-Season Athlete
Now you can ask, from the list of athletes, who has participated in both Winter and Summer Olympic Games?
// read query
def multi_season_athlete(person) {
exists(g1, g2:
OlympicGraph:participates_in(person, g1)
and OlympicGraph:has_type(g1, ^GameType["Winter"])
and OlympicGraph:participates_in(person, g2)
and OlympicGraph:has_type(g2, ^GameType["Summer"])
)
}
def output(person, person_name) {
multi_season_athlete(person)
and OlympicGraph:has_name(person, person_name)
}
You get one result back and you see that Eddy Alvarez is the most diverse athlete. He has participated in both Winter and Summer Olympic Games (Sochi in 2014 and Tokyo in 2020). Here’s how:
The code above defines the relation multi_season_athlete
.
This returns those athletes, if any, who have participated in both Summer and Winter Olympic Games.
The query is a little more complex than examples you have seen so far. It’s a navigational query, as it traverses a graph, finding the athletes from the connections you specify.
The variable g1
refers to Olympic Games with the value ^GameType["Winter"]
and g2
refers similarly to Olympic Games with the value ^GameType["Summer"
].
You are asking the system to find you the athlete(s) who participated_in (participates_in
) both g1
and g2
Games — that is, athletes who have participated in both Winter and Summer Olympic Games.
The exists
part of the query specifies that two games need to exist that fulfill the subsequent condition, but that you don’t care which ones they are.
In technical terms, the variables g1
and g2
are existentially quantified.
By creating and querying your knowledge graph, you have analyzed your data and found the most successful athlete according to your chosen definitions of success. Allyson Felix is the most successful when success is defined as the number of Olympic Games an athlete participates in. If success is defined by diversity of Olympic Game types, Eddy Alvarez is the most successful athlete.
At a later stage, as you fully test out more complex definitions, you can add them to your model. This way, they can be saved in the database and shared across all your queries. This is how you extend the knowledge graph with new information, building up a semantic layer on top of your base data. See the test queries in The Lehigh University Benchmark for examples.
Visualizing Your Data Graph in Rel
Throughout this tutorial you have been using ORM diagrams to get a schema-level (for example, Olympics
, Athlete
) visualization of the data as it is a simple yet efficient way of looking at it.
Rel allows you to get a data-level (for example, Tokyo 2020
, Eduardo Cortes Alvarez
) visual representation of it.
Here is how to build this visualization using graphviz:
// read query
// Define the graph relation.
module DataGraph
with OlympicGraph use Athlete, Olympics, participates_in, has_name
def node(name) {
exists(e :
(Athlete(e) or Olympics(e))
and name = string[has_name[e]]
)
}
def edge(name1, name2) {
exists(e1, e2:
participates_in(e1, e2)
and name1 = string[has_name[e1]]
and name2 = string[has_name[e2]]
)
}
end
// Plot it.
def output = ::std::display::graphviz[DataGraph]
Above is the Graphviz representation of a section of your data.
Here you are using a module again, called DataGraph
, within which you will define the graph relations.
First you state that within the DataGraph
module you are using Athlete
, Olympics
, participates_in
, and has_name
, which are all stored in the OlympicGraph
base relation.
Then you define that Athlete
and Olympics
entities make up the nodes and participates_in
is the only edge between those nodes that should be displayed.
As you have seen in previous queries, has_name
is required in order to display the names of the Olympic Games and of the athletes.
Before passing the data to Graphviz, the value type data is converted to a String with string
.
Summary
This tutorial has introduced you to the concept of knowledge graphs, entity nodes, value nodes, and edges. You have learned how to model relationships between pieces of data, and how to express these relationships in Rel in the form of a knowledge graph. You also learned how to translate questions into graph queries in Rel that reason over your knowledge graph. You can learn more about those concepts as well as how to build and query a relational knowledge graph in Elements of a Relational Knowledge Graph.
Expressing queries across interesting, complex, knowledge graphs is what Rel does best. You can find a concrete example of that in the Lehigh University Benchmark how-to guide.